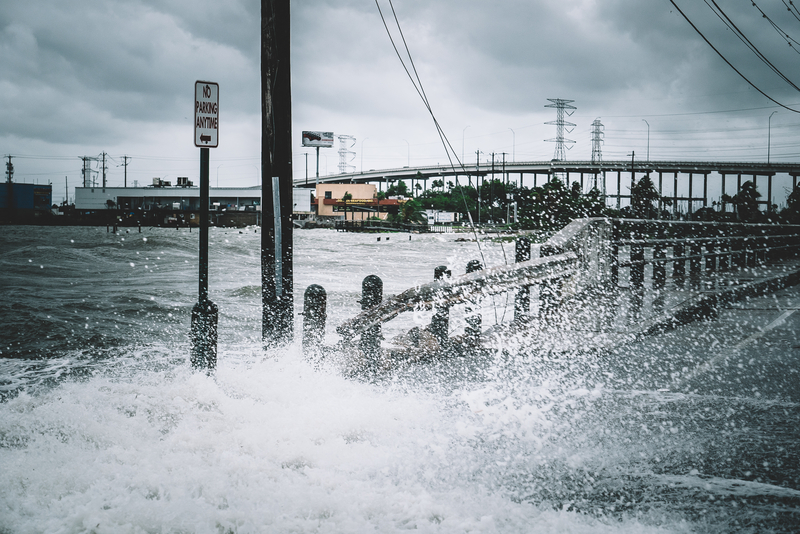
Hurricane Harvey was the first category 4 hurricane to hit Texas since 1961 and caused widespread power outages in August 2017. Credit: Eric Overton|Dreamstime.com.
Protecting our decades-old infrastructure against the growing frequency of extreme weather events has become a top priority. But it can be a daunting task when that infrastructure is as complex as the U.S. power grid: Its 12,000 utility-scale power plants use 160,000 miles of high-voltage transmission lines—and many more low-voltage lines—to deliver electricity to our homes and businesses.
To make the grid more resilient, a good start is to locate its weakest spots, or those most strongly affected by past extreme events. An example is Hurricane Harvey, which hit Texas in August 2017. To examine its impact on the state’s power grid, AAE professor Sheldon (Xiaodong) Du teamed up with two former graduate students: Yue Zhao M.S.’19 and Adria Brooks PhD’20, who worked on the study while earning her PhD in electrical and computer engineering.
The chosen example had some advantages. “The movement of hurricanes is easier to track than floods or wildfires, and the Texas power grid is electrically isolated from the country’s Eastern and Western grids,” says Du.
Economists like to analyze prices because they measure the relationship between supply and demand in a market of interest, say the housing or consumer goods market. They also capture the response to sudden changes in that relationship due to natural disasters. That’s why Du used electricity prices—obtained from the Electric Reliability Council of Texas (ERCOT)—to define the grid’s weakest spots as those with the largest price response. But electricity is a unique commodity, and understanding the drivers of its cost was a critical first step.
Electricity is traded in the state’s competitive wholesale electricity market, where sellers are generators and buyers are large users and utilities (which sell their purchased electricity to homes and businesses on the retail market.) Grid operators at more than 600 “pricing nodes” determine electricity prices by balancing local supply and demand.
These operators are similar to stockbrokers, but the energy market has some distinct features. For example, once electricity has been generated, it cannot easily be stored. Thus, local market clearing prices are set by matching total supply to total demand at regular intervals. All generators then charge the same clearing price to all utilities to whom they send electricity.
Another distinct feature is the limited capacity of transmission lines. These high-voltage lines connect generators to substations and can be up to 300 miles long. Substation managers reduce the voltage before sending electricity to utilities on shorter distribution lines. The material and age of transmission lines determine how much electricity they can carry without failing.
When a low-cost generator’s line fails due to congestion, operators need to switch to other, more expensive generators to meet the current demand. Think of taking a longer route to the grocery store when the shorter route has a traffic jam; the higher price is the longer travel time.
The nature of this wholesale market explains why local clearing prices measure the grid’s ability to meet the local demand for electricity—both in normal times and during extreme events. In the case of Hurricane Harvey, the researchers analyzed 139 pricing nodes with known geographic locations in four load zones, or electricity consumption areas.
“Modeling the relationship between average hourly prices at these 139 nodes and changes in the hurricane’s path provided a unique opportunity to study the economic impact of a natural disaster on the Texas power grid at a high level of detail,” says Du.
The team used space and time information to create two comparison groups. Since Harvey’s path crossed the southern part of Texas, 68 nodes in the South and Houston load zones made up the “treatment” and 71 nodes in the West and North zones the “control” group. The researchers defined the treatment period as 125 hours between 7 pm on August 25 (30 minutes before the first reported power outage) to 12 am on August 30, after Harvey’s last landfall in Louisiana. The control period included 125 hours up until 6:59 pm on August 25.
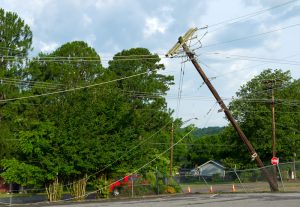
The statistical analysis of treatment and control data predicted counterfactual prices at the 68 treatment nodes, or the most likely market prices if Harvey had not hit Texas. The team identified the 21 most vulnerable nodes as those where observed prices were significantly higher than their counterfactuals.
One reason for the greater resilience of the other 47 nodes was their longer electrical distance from Harvey’s center in terms of transmission lines. The 21 most vulnerable nodes were also connected to significantly fewer nodes that could serve as backups, highlighting the importance of network connectivity.
An analysis of the 31 counties containing the 68 nodes showed that low-income counties and those exposed to more hurricanes between 1953 and 2016 experienced significantly greater price shocks. The federal government includes similar measures of “social vulnerability” in its national risk index for hurricanes and other environmental hazards. This index ranks counties on a scale from 0 to 100 to help agencies develop targeted hazard mitigation strategies.
Knowing the location of vulnerable nodes can help prioritize efforts to improve grid resilience, says Du. Such efforts range from building new or upgrading existing transmission lines to using grid-enhancing technology or adding autonomous microgrids, which can disconnect from the main grid to supply backup electricity in high-risk areas during extended outages.
“Natural disasters are always costly and tragic, but they also teach us important lessons about how to adapt to change and be better prepared for the future,” says Du.