Siming Yan
M.S. Agricultural and Applied Economics-Professional Option (MSPO)
- Hometown
- Shanxi, China
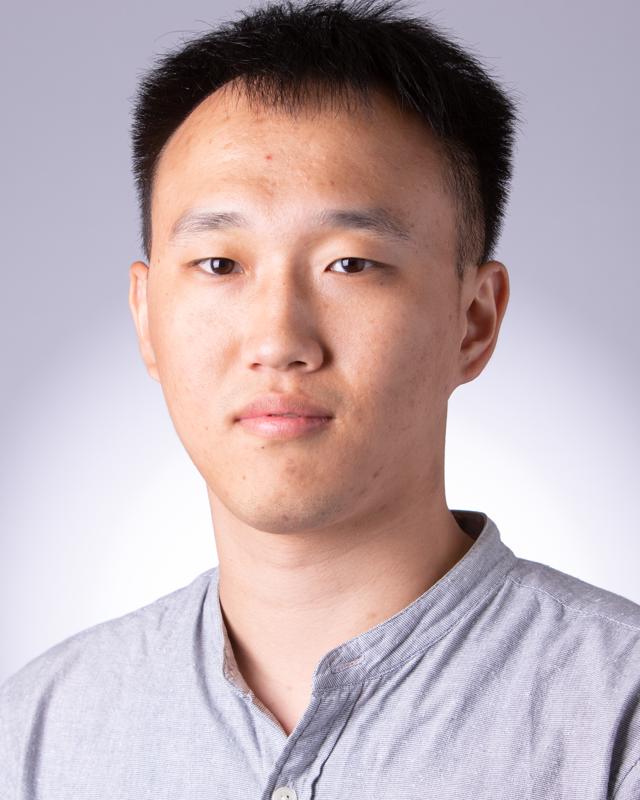
Bio Sketch
During one and a half years of training at the University of Wisconsin-Madison, I developed a good understanding of econometric analysis and machine learning methods. My interest in data analysis has grown through my experience in learning from the courses. During my first semester, I developed several models with fixed effects and clustered errors, therefore evaluated the econometric impact of 68,000 households’ energy-consuming behavioral response to the implementation of energy-saving programs. This study is built upon a random controlled trial and I obtained statistically significant results in the interest variables to prove the effectiveness of such program implement. My interests then went to machine learning. In the following semester, I used machine learning techniques such as *Support Vector Machine*, *Random Forests* and *Stochastic Gradient Boosting Machine* to predict used car price in the India market from the year 1996 to 2019, and also identified causal inference by using *Ordinary Least Squares*, *Orthogonal Machine Learning*, *LTE Lasso Regression*, *Causal Tree* and *Causal Forests*. My results showed *Stochastic Gradient Boosting Machine* generated best predictions with lowest *root mean square error*, followed with *Random Forests* and *SVM*. Currently, I finally have a chance to manipulate my own data of power plant monthly electricity-generating data in Shanxi province, China, from December 2016 to June 2020. My data includes 485 power plants in Shanxi with four different types of fossil-fuel, hydro, wind, and photovoltaic. I used my data set to answer three questions that I am interested in:
During one and a half years of training at the University of Wisconsin-Madison, I developed a good understanding of econometric analysis and machine learning methods. My interest in data analysis has grown through my experience in learning from the courses. During my first semester, I developed several models with fixed effects and clustered errors, therefore evaluated the econometric impact of 68,000 households’ energy-consuming behavioral response to the implementation of energy-saving programs. This study is built upon a random controlled trial and I obtained statistically significant results in the interest variables to prove the effectiveness of such program implement. My interests then went to machine learning. In the following semester, I used machine learning techniques such as *Support Vector Machine*, *Random Forests* and *Stochastic Gradient Boosting Machine* to predict used car price in the India market from the year 1996 to 2019, and also identified causal inference by using *Ordinary Least Squares*, *Orthogonal Machine Learning*, *LTE Lasso Regression*, *Causal Tree* and *Causal Forests*. My results showed *Stochastic Gradient Boosting Machine* generated best predictions with lowest *root mean square error*, followed with *Random Forests* and *SVM*. Currently, I finally have a chance to manipulate my own data of power plant monthly electricity-generating data in Shanxi province, China, from December 2016 to June 2020. My data includes 485 power plants in Shanxi with four different types of fossil-fuel, hydro, wind, and photovoltaic. I used my data set to answer three questions that I am interested in:
Personal Reflection
Graduation is one of the most important events in everyone's life. It is a mark of growth, a trophy of personal development. I love UW-Madison who gave me so many opportunities to improve myself. I appreciate the professors who helped me during the past 18 months.
Graduation is one of the most important events in everyone's life. It is a mark of growth, a trophy of personal development. I love UW-Madison who gave me so many opportunities to improve myself. I appreciate the professors who helped me during the past 18 months.